Online only event - You must pre-register to receive access information.
You must be logged in to register.
Speaker: Richard Gibson, NanoSeis
Co-Author: Ben Schaeffer, NanoSeis
Distributed acoustic sensing (DAS) data provide important insights into hydraulic fracturing programs, and applications include characterization of developing fracture systems as well as operational issues related to casing integrity and plug performance. One advantage of DAS is that the fiber optic cable provides continuous measurements of strain and strain-rate signals along the entire length of the borehole with very high spatial and temporal sampling rates, providing a very rich - but high volume - data set. Low-frequency (i.e., less than 1 Hz) content provides constraints on fracture propagation from a treatment well to nearby monitor wells, while high frequencies (~100 Hz) allow detection and location of microseismic events to map fracture propagation in the interwell space. This provides an ideal case for the application of machine learning techniques: huge data volumes, with results depending on analysis of features with broad ranges of scales in space and time.
In this talk, we will discuss several examples of scientific machine learning (SciML) techniques to address these problems. Given the rapid delivery of large data volumes, automatic detection of microseismic events is essential. Convolutional Neural Networks are well suited in this instance, as they are highly effective at producing a non-linear statistical representation of a tuned set of physics driven engineered features. This approach, however, is dependent on the quantity and quality of the training data; thus, development of synthetic data is crucial. The synthetic training data used for initial training of the networks include simulations applying realistic models of seismic anisotropy and its impact on source radiation to develop reliable results, and we also applySciML techniques for data-driven extraction of spatiotemporal analysis of data and inversion of fracture strain signals. Finally, we note that modern automatic differentiation (AD) tools can play an important role in the implementation of efficient and accurate models for simulation and interpretation of fracture-related signals. We will present several examples of these approaches to demonstrate the value of SciML for analysis of DAS data.
Speaker Biography: Dr. Rick Gibson, Chief Geophysicist, NanoSeis
Rick Gibson is Chief Geophysicist for NanoSeis, focusing on microseismic data analysis, modeling, inversion, and exploring how AI technologies could be incorporated into standard workflows. Prior to joining NanoSeis, Rick had a 22-year long and distinguished career at Texas A&M University where he was a Professor of Geophysics and Associate Director of the Berg-Hughes Center for Petroleum and Sedimentary Systems. Together with the many students he supervised over the years, his research concentrated on understanding elastic wave propagation in complex media and the application of that understanding to solving practical problems in hydrocarbon exploration, reservoir monitoring, and crustal seismology. Numerical modeling and computational aspects therefore played a central role in Rick's research interests. These efforts regularly led to interdisciplinary collaborations with colleagues in petroleum engineering, mathematics, and other branches of engineering. Rick has published numerous papers in his fields of expertise. Rick holds a PhD from the Massachusetts Institute of Technology and has done postdoctoral research at the Université Joseph Fourier in Grenoble, France, and MIT in Cambridge, Massachusetts.
** Access information will be sent to all registrants after registration closes.
THANK YOU TO OUR SPONSOR:
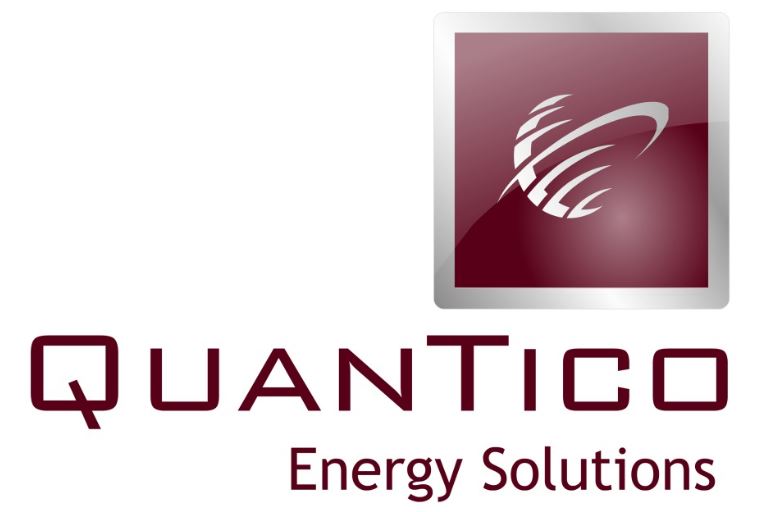